Unlocking Business Success:
The Power of Data Observability
Data plays a pivotal role in decision-making. Knowing the antecedents of data requires you to keep data well-conditioned. Data further helps reg-tech in ensuring organizations meet BCBS239, SOX, GDPR, and other guidelines. Observing the health and performance of Data is no longer optional, it’s a must in modern enterprises.
Traditionally, Data Observability has relied on three foundational pillars: operations logs, metrics, and traces. Most tools available in the market have historically focused on these aspects. However, this field is rapidly evolving and expanding its horizons, particularly with the introduction of active metadata.
As applications become more distributed across containers or cloud environments, it becomes difficult to observe the entirety of your system using only traditional tracking methods. A better approach is to use observability solutions to centralize and display metrics and logs from across the systems and monitor any drifts.
As a result, Data Observability is progressing toward providing organizations with a comprehensive understanding of the health and performance of their data, data pipelines, data landscapes, and data infrastructure. It empowers organizations to gain a comprehensive understanding of the health of their data ecosystem, covering critical aspects like:
- Data Integrity
- Data pipelines’ reliability
- The robustness of data infrastructure
- Data Users
- Financial Governance
This ecosystem embodies various dimensions, including applications, data, control, and infrastructure. Any of these dimensions can potentially experience a drift, and observability plays a pivotal role in identifying these deviations. These drifts can manifest in various forms, such as discrepancies in code, data, metadata, technology, configuration, schema, or infrastructure.
Advantages of Data Observability Throughout the Data Ecosystem
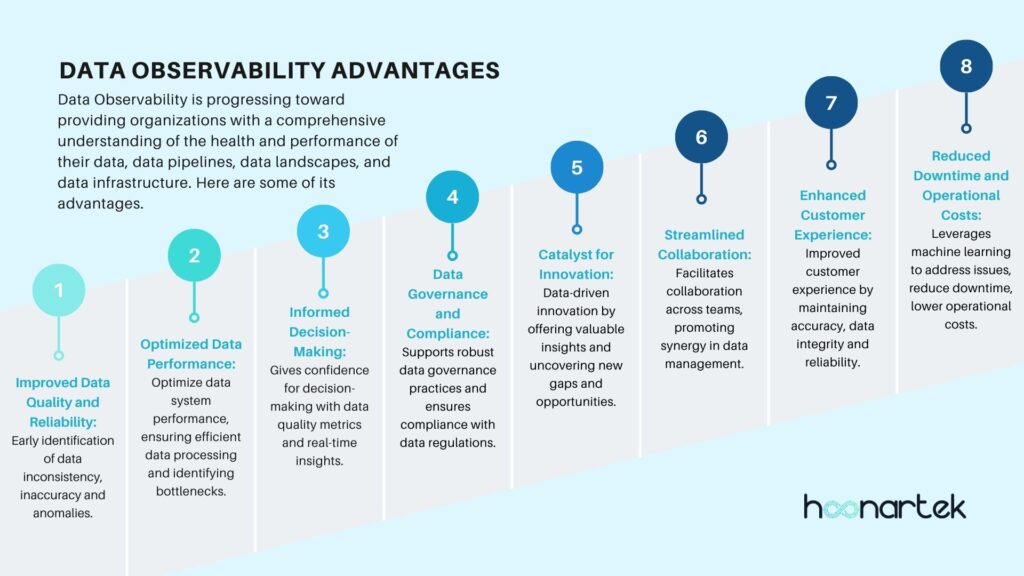
Improved Data Quality and Reliability:
- Early identification of data inconsistency, inaccuracy and anomalies which leads to improved data quality and reliability is critical for data-driven operations.
Optimized Data Performance:
- Help optimize data system performance, ensuring efficient data processing by identifying the bottlenecks early on.
Informed Decision-Making:
- It provides confidence for proactive decision-making by virtue of known data quality metrics and real-time insights into the data ecosystem’s health.
Data Governance and Compliance:
- Supports robust data governance practices and ensures compliance with data regulations by the visibility into how data is collected, processed, and used.
Catalyst for Innovation:
- Fosters data-driven innovation by offering valuable insights and uncovering new gaps and opportunities through exploration.
Streamlined Collaboration:
- Facilitates collaboration across teams, promoting synergy in data management.
Enhanced Customer Experience:
- Contributes to an improved customer experience by maintaining accuracy, data integrity and reliability.
Reduced Downtime and Operational Costs:
- Leverages machine learning for end-to-end monitoring, identifying data issues and root causes effectively. By proactively addressing issues, minimizes downtime and lowers operational expenses.
Metadata serves as tangible evidence of a data’s journey and usage. Many organizations possess substantial metadata resources, yet often, they remain scattered and underutilized. It’s time to take a systematic approach by initiating an inventory and cataloging process.
The Approach:
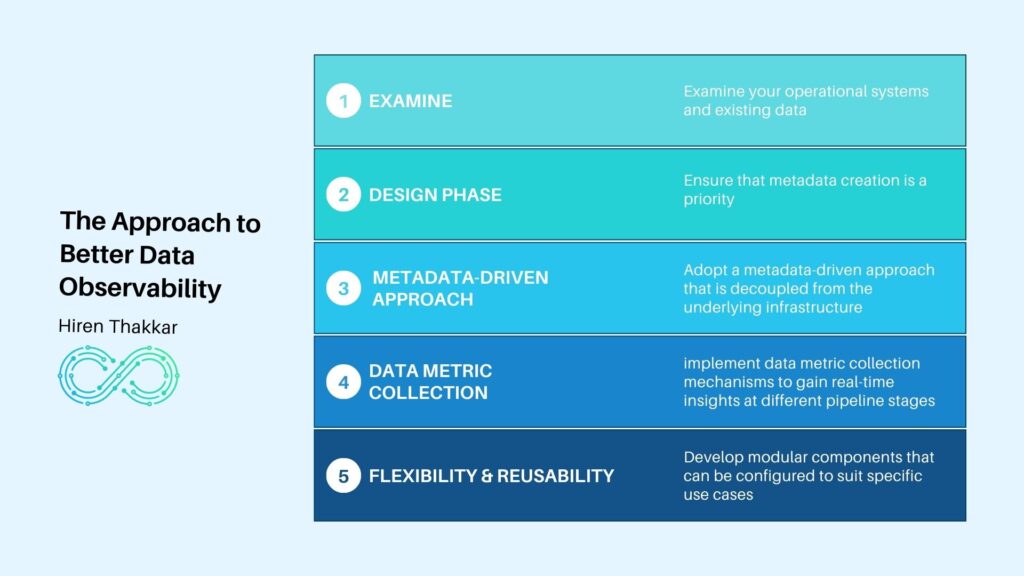
Begin by examining your operational systems and existing data. These systems provide an initial repository of metadata.
During the design phase of any data-related project, ensure that metadata creation is a priority. Identify gaps in your metadata repository and take proactive steps to fill them.
For future data pipelines, adopt a metadata-driven approach that is decoupled from the underlying infrastructure. Additionally, consider implementing data metric collection mechanisms to gain real-time insights at different pipeline stages.
To enhance flexibility and reusability, develop modular components that can be configured to suit specific use cases.
The following are the most important best practices for an efficient implementation of Data Observability:
Observing Data:
- Implement Enterprise-Wide Standard Rules and Practices for Data Quality Metrics Collection and Reporting
- Define Appropriate Thresholds for data drifts with alerting mechanisms
Observing Data Pipelines:
- Perform Impact Analysis prior to any change
- Detect Volume Anomalies
- Identify Performance Anomalies
- Capture Real-Time Data Metrics at the Pipeline and Stage Levels
Observing Infrastructure:
- Set Precise Thresholds for each metric of all infra-resources
- Monitor Design vs. Actual Usage Drift
Observing Data Users:
- Monitor Role-Based Access Control (RBAC) and Attribute-Based Access Control (ABAC) Change Logs
- Establish Alerting Mechanisms for Changes
- Who owns and modifies data?
- Who uses data and their access frequency?
Observing Cost and Financial Impacts:
- Identify Data Set Costs and Resource Utilization Costs
- Establish Budgets, Usage Limits and use for capacity planning
- Conduct Regular Financial Reviews (or create a dashboard)
- Financial impacts of SLA breaches
- Establish the financial impact due to system downtime
Monitoring and Alerting Drifts:
- Ensure Timely Alerts
- Establish Resilience Measures to Mitigate Data Drift, Code Drift, and Technology Drift
By refining these practices, organizations can enhance their data management, maintain data quality, and effectively monitor their data ecosystem while also ensuring financial prudence.
Data Environment Considerations
Modern Data Observability tools employ a range of advanced techniques, including automated monitoring, root cause analysis, tracking of data lineage, data catalogs, knowledge graphs and data health insights. These tools enable organizations to proactively identify, address, and even prevent data anomalies or downtime by enabling self-healing pipelines. This proactive approach yields a variety of benefits, including more robust data pipelines, improved team collaboration, enhanced data management practices, and ultimately, a higher level of customer satisfaction.
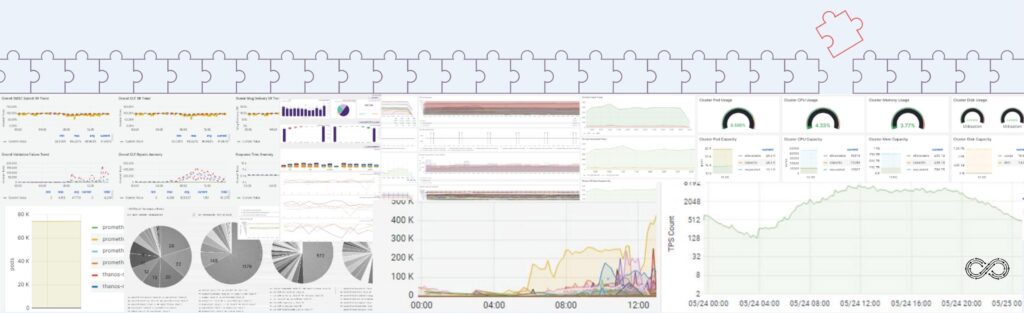
Hoonartek has been ensuring for years that all the delivery includes hooks to enhance enterprise data observability. We have enabled clients on various data observability tools like #Splunk #Prometheus, #Elastic, #Grafana, #Kibana, #AWS #Cloudwatch, #DataDog, #OpenTelemetry, #FluentBit and #InfluxDB to name a few.
Data Observability Now
Planning or Working on Data Observability initiatives? Talk to a seasoned team of senior architects, solutions specialists, and data leaders for your data observability needs.
In a crowded marketplace, it can become daunting to choose the right solution for your overall Data Observability needs. In our assessment, Ab Inito provides the best coverage – especially in bulk/batch data processing, data federation, quality of training and support over its competition.
Ab Initio provides a control center with the real-time integrated job and system data overlaid. This information is stored in the database with trends. It offers plans and graphs to generate job tracking metrics, custom metrics that you define, and logs. Ab Initio also provides deep tracking of metrics by embedding them in the function call at any point in the process. It supports pull as well as push methods to publish these metrics and logs to the tool of your choice whether it is on-prem or third-party cloud-native solutions, such as Prometheus or AWS CloudWatch. It is easier to write custom metrics particular to your applications, thresholds and events that conform to the open metrics specifications.
Metrics and logs offer a more complete picture of your application across the distributed applications. Logs can bring insights into granular information on events and metrics can provide the overall health of the system over the period and trends which helps identify the anomalies.
Final word
Data Observability is a powerful toolbox that can arm your teams with a comprehensive understanding of the health and performance of your data ecology. Deployed correctly, it will futureproof your data ecosystems, help prevent anomalies, and discover unknowns in data ecosystem.
About the Author
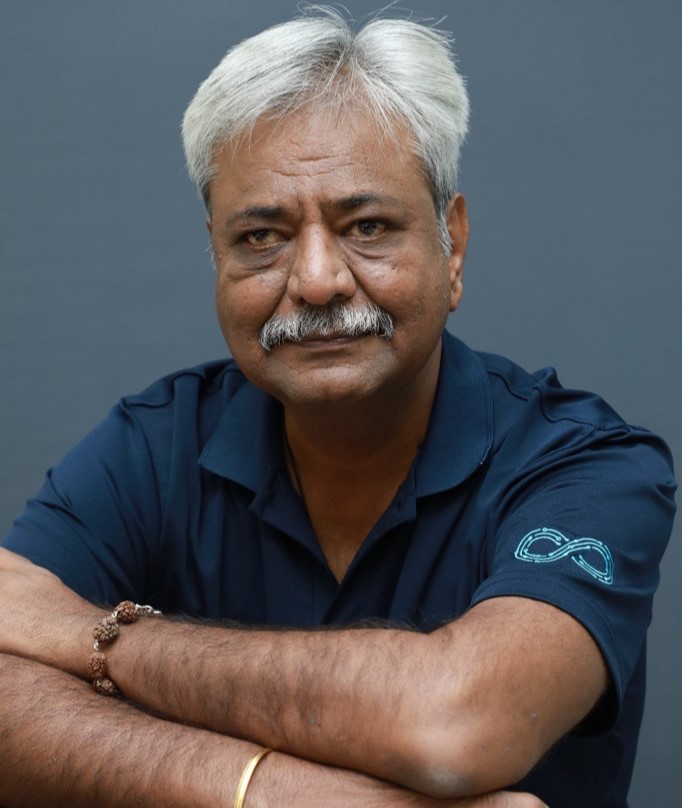
Hiren Thakkar
Hiren Thakkar, a seasoned technologist, is renowned for his expertise in crafting high-performance data platforms for Fortune 100 companies. His prowess spans data integration, data warehousing, data monetisation using varied technologies. Embracing the Kaizen philosophy, Hiren instils a culture of continuous improvement, fostering innovation within his team. Connect with him on LinkedIn.