Navigating the Landscape of Data Quality
Data is a key factor in every aspect of a business. Everything revolves around it. Organizations store, manage and use large amounts of data daily. In the world of business, data is like the puzzle pieces that help us make decisions. But just having the pieces isn’t enough – they need to fit perfectly. That’s where data quality comes into the picture. The quality of data is paramount.
Businesses and organizations rely on data for making critical decisions, devising strategies, and delivering exceptional customer experiences.
Decision makers lose confidence when there is missing information, duplicate information, and data inconsistency. The best way to build confidence in enterprise data is to measure, correct, and monitor data quality across the enterprise. Hence the data quality becomes the first candidate when data observability is considered. Any drift in data quality needs to be observed. But what exactly is data quality, why does it matter so much, and how can we ensure that the data we use is of the highest quality?
This blog post explores the concept of data quality, its significance, the consequences of poor data quality, and effective strategies for rectifying and enhancing data quality.
What is Data Quality?
Data quality refers to the overall condition or suitability of data for its intended use. It is a measure of how well data meets certain criteria or requirements, including accuracy, completeness, consistency, reliability, and timeliness.
What is Poor Data Quality?
Poor data quality refers to data that is inaccurate, incomplete, inconsistent, outdated, or otherwise unreliable for its intended purpose. Bad data is like a broken compass; it points you in the wrong direction. This occurs when data lacks accuracy, consistency, or reliability, leading to incorrect conclusions and misguided decisions.
It can have significant negative impacts on businesses, research, decision-making, and various other applications that rely on accurate and dependable data. Ensuring data accuracy requires ongoing effort. Implementing data quality checks, utilizing automated tools, and fostering a data-conscious culture within your organization are crucial steps toward minimizing poor data quality’s impact.
As per the Global data management research report by Experian, around 95% of businesses have seen negative impacts to the business due to poor data quality. These effects range from negative customer experiences to a loss of customer trust.
Why Does Data Quality Matter?
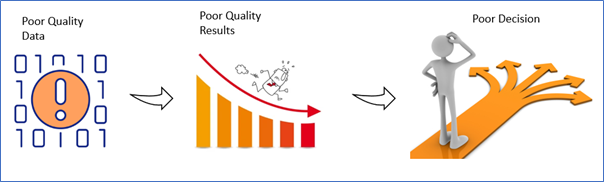
Data quality is important for a variety of reasons, as it directly impacts the effectiveness of decision-making, business operations, research, and overall organizational success.
Poor data can have a significant impact on important business projects. It impacts every aspect of your business ranging from sales and marketing to customer service and support.
Here are several key reasons why data quality matters
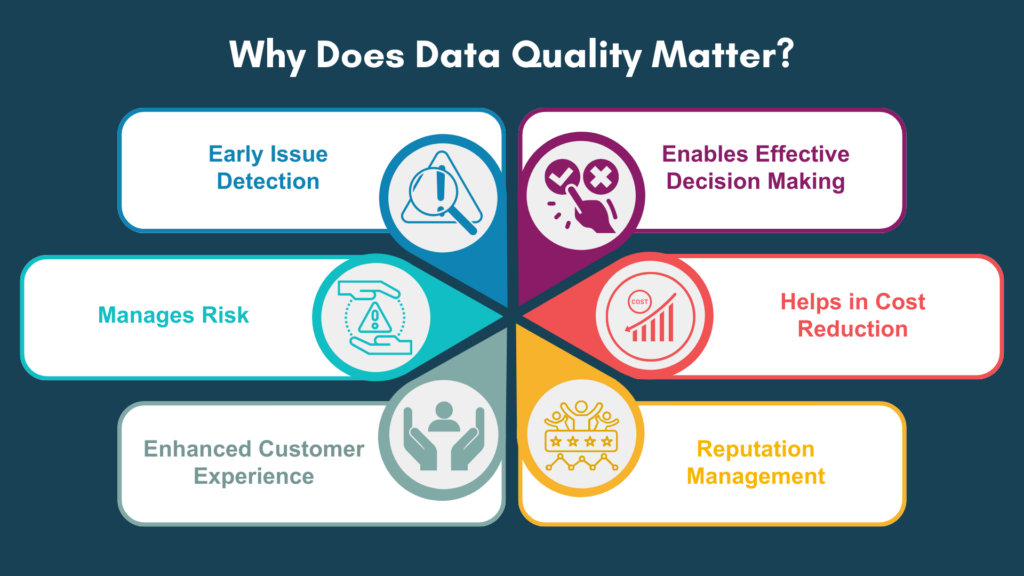
Effective Decision Making
High-quality data is essential for making informed and reliable decisions. Businesses and researchers rely on trustworthy data to draw accurate conclusions and make valid recommendations. Poor data quality can lead to misguided decisions, which can have serious consequences for businesses. According to a Forbes report, 84% of CEOs are concerned about the quality of their data.
Cost Reduction
Correcting errors caused by poor data can be costly and time-consuming. Investing in data quality upfront can help avoid these costs. As per Gartner in 2021, poor data quality cost organizations an average of $12.9 million each year. As per IBM’s estimate the yearly cost of poor-quality data in the US alone, in 2016 was $3.1 trillion.
Reputation Management
Poor data quality can damage a business/organization’s reputation. Businesses with bad data may struggle to maintain customer trust and satisfaction, which can lead to harm to their reputation and a decline in business.
Enhanced Customer Experience
High-quality data helps you understand your customers better. This knowledge enables personalized interactions, targeted marketing, and improved customer satisfaction, ultimately fostering stronger customer relationships.
Risk Management
High-quality data helps organizations identify and manage risks effectively, reducing the chances of unexpected challenges. Overall, the cost of incorrect data can be substantial and negatively impact an organization’s bottom line. That is why businesses need to prioritize data quality and put a process in place to make sure the data is accurate, and consistent.
Early Issue Detection
Regular data profiling and monitoring allow you to identify data quality problems early, minimizing the impact on your operations and decision-making.
Need help to optimise your Data Quality?
Unlock the power of your data with Hoonartek’s expert Data Quality services and go from insights to action faster.
How Data Quality Is Generated
Data quality is generated through a combination of careful planning, diligent data collection, validation processes, and ongoing maintenance efforts. Here’s a simplified overview of how data quality is generated:
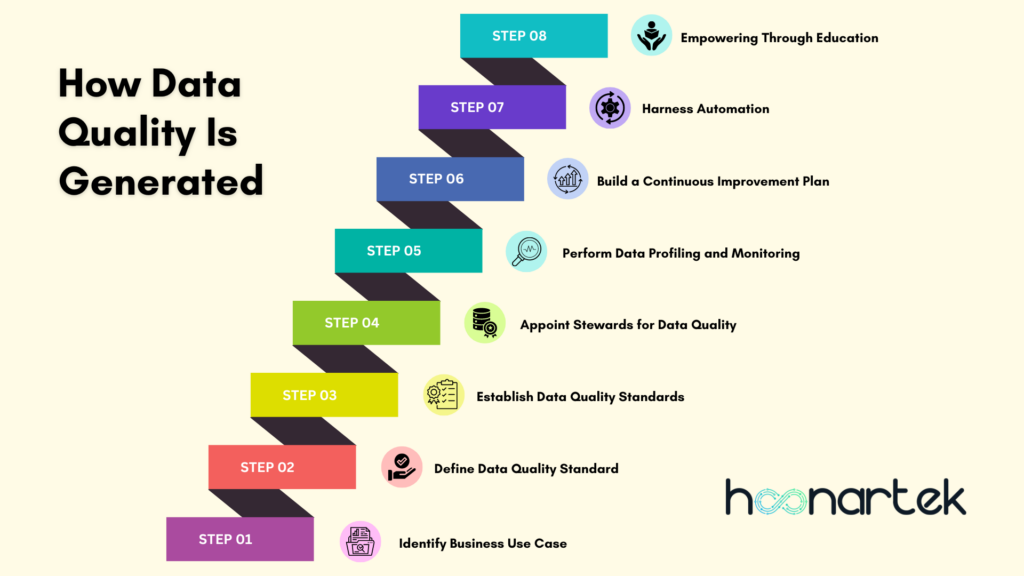
Identify Business Use Case
Identify the datasets that are directly tied to critical processes, decisions, and customer experiences. Gain a clear understanding of your Key Performance Indicators (KPIs) and Key Risk Indicators (KRIs). These metrics are crucial for measuring business success and identifying potential risks. Align your data quality improvement efforts with the data that feeds into these indicators. Create a compelling business case by identifying the “hot spots” where data quality improvements can have the most significant positive impact.
Cost Reduction
Correcting errors caused by poor data can be costly and time-consuming. Investing in data quality upfront can help avoid these costs. As per Gartner in 2021, poor data quality cost organizations an average of $12.9 million each year. As per IBM’s estimate the yearly cost of poor-quality data in the US alone, in 2016 was $3.1 trillion.
Establish Data Quality Standards
Begin by aligning data quality standards with regulatory and compliance requirements relevant to your industry.
Ensure data quality standards directly contribute to achieving strategic objectives. Implement a recurring review process for effective data quality standards. Aim for a “good enough” level of data quality that supports informed decision-making and business processes effectively.
Appoint Stewards for Data Quality
To effectively manage data quality, appoint dedicated data stewards and foster collaboration across business units and the Data & Analytics (D&A) team. Ensure that data stewards are accountable for maintaining and enhancing data quality.
Perform Data Profiling and Monitoring
Perform regular data profiling and monitoring as an ongoing process to ensure data quality and identify potential issues. Identify the critical data elements that are essential for your business processes and measure data quality on those elements.
Define data quality metrics for each element, such as accuracy, completeness, consistency, and validity. Use data profiling tools to examine the selected data elements. Set up automated monitoring processes that continuously evaluate the quality of the critical data elements.
Implement alerts or notifications for any sudden changes, anomalies, or outliers in the data. This proactive approach helps you identify and address issues in real time. Establish baseline data quality metrics and benchmarks for each targeted data element. Regularly measure and compare the current data quality against these benchmarks. This helps you track the progress of your data quality improvement efforts and identify areas that need more attention.
Build a Continuous Improvement Plan
Build an improvement plan based on the insights gained from data profiling and analysis by collaborating with data stewards and relevant stakeholders. Understand why inconsistencies are occurring  and based on root cause analysis build a cleansing plan. Document all actions and learnings. This will help to understand what worked and what didn’t, which can inform future data quality initiatives.
Harness Automation
Automate data quality initiative. Automation plays a pivotal role in maintaining data quality. Automated processes ensure consistent data quality across the organization.
Empowering Through Education
Incorporating a data quality (DQ) mindset and best practices into data literacy programs is a strategic approach to foster a data-driven culture that values and prioritizes data quality. Educate employees about the impact of poor data quality on decision-making, operational efficiency, and customer satisfaction.
Emphasize that data quality is everyone’s responsibility, regardless of their role. Encourage employees to take ownership of the data they work with and to report any data anomalies or issues they encounter.
Data Quality Dimensions
Data quality dimensions are criteria that we define to assess data quality. Focusing on data quality dimensions helps organizations ensure that their data is trustworthy, actionable, and aligned with their business goals. There are six core data quality metrics as given below:
Accuracy
Accuracy measures the correctness of data. Inaccurate data is detrimental to business as it erodes trust in the data. Example: If a customer’s name is Mark Brown, but the system displays it as Mark Brawn, which is inaccurate.
Completeness
Completeness measures the availability of required data attributes. A complete dataset should contain all the necessary attributes. Incomplete information can render the dataset unusable. Example: If an organization seeks customers over the age of 40 and relies on birthdate information, but the birthdate attribute is empty, it means the data is incomplete.
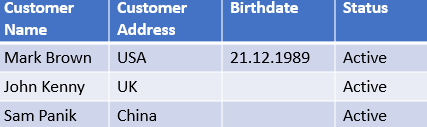
Consistency
Consistency measures whether data stored in one location matches the same data stored elsewhere. Consistency ensures there are no conflicts between identical data values in different places. Inconsistent data undermines confidence in the data. Example: If an HR system indicates that an employee has left the organization, while the payroll system shows the employee as active, it means data is inconsistent.
HR System Data
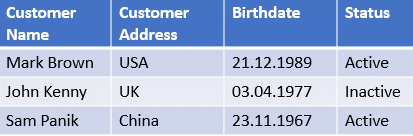
Payroll System Data
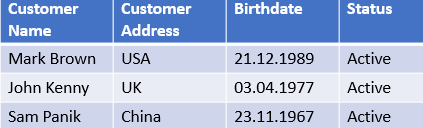
Timeliness
Timeliness measures the availability of updated data when needed. Timely data is updated to remain current and accessible when required. Example: If management requires a weekly status report every Friday, but the report isn’t ready on time, the data does not meet users’ expectations.
Uniqueness
Uniqueness measures the absence of duplicate records or attributes. Uniqueness ensures that no data is recorded more than once. Example: If multiple records exist for a person named Mark Brown, born on 21.12.1989, and residing in the USA, there are duplicate entries.
Validity
Validity measures data against specific formats or business rules. Valid data contains appropriately structured values. Example: If the birthdate attribute is supposed to be in DD/MM/YYYY format, but a user enters it as MM.DD.YYYY, the data is invalid.
Conclusion
In conclusion, data quality is the measure of how accurate, complete, consistent, and reliable data is within an organization. The quality of data matters because it directly impacts decision-making, operational efficiency, and customer satisfaction. Tool and technology might change but adopting a data quality culture is also important.
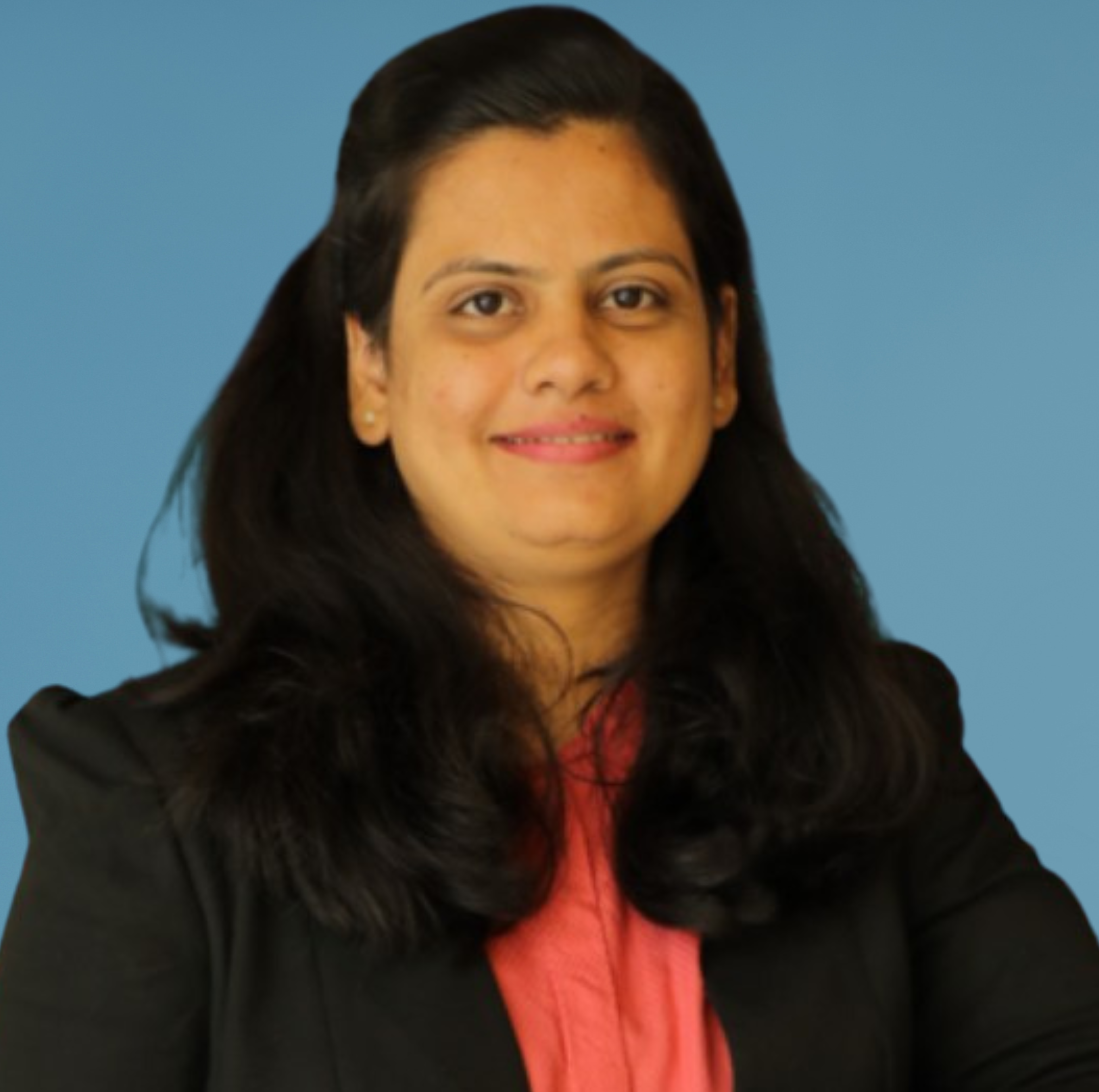
Rasika Salunkhe
Rasika is a data management professional with a passion for leveraging the Ab Initio Metadata Hub to drive successful outcomes. She specializes in using the Hub's capabilities to ensure high data quality, accurate business lineage, and efficient data discovery. Rasika's expertise lies in Data Lineage and Dependency Analysis, Data Integration, Metadata Hub Functionality, Data Catalog Implementation and Ab Initio Testing Framework.​